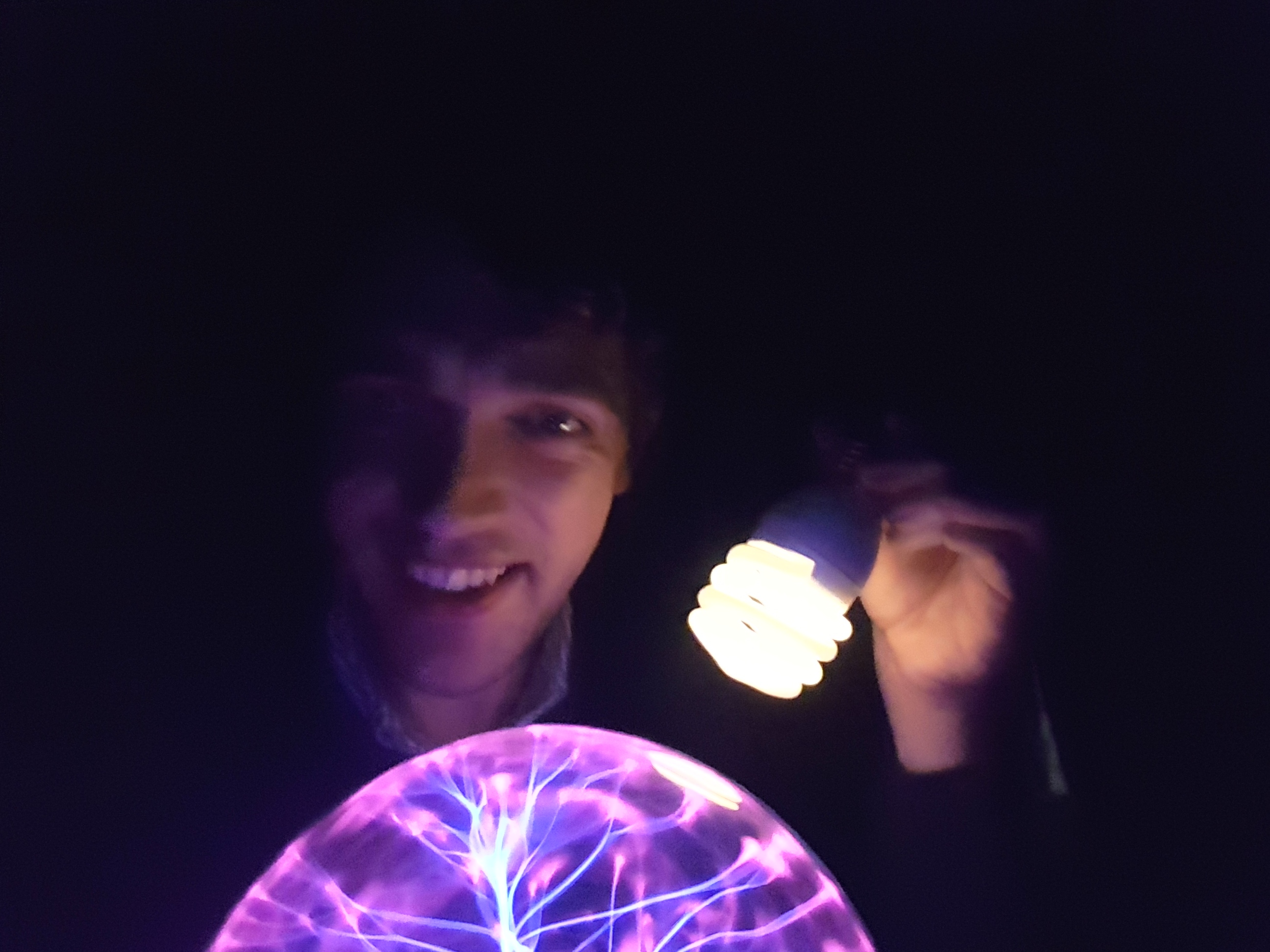
Dr Arthur Turrell is an economic data scientist working in the public sector. He is the former Deputy Director and Acting Director of the ONS Data Science Campus. He currently works at the Bank of England. He started his career in physics and remains a visitor to the plasma physics group at Imperial College London. His research covers data science, economics, and physics.
As well as research, Arthur is passionate about making science and economics more accessible — mostly because scientists shouldn’t be allowed to have all the fun. You can find more information about his outreach activities and links to some of his writing on the media page, while information on The Star Builders, his popular science book on nuclear fusion, can be found here.
Arthur is a Royal Statistical Society William Guy Lecturer for 2025-2026, and his lecture is on “Economic statistics and stupidly smart AI.” There will be a link to the lecture in the media section once it is available.
While previously at the Bank of England, Arthur was lucky enough to be asked to help to design a new bank note featuring Alan Turing. Arthur chose the mathematical elements from Turing’s work to feature on the note. If you’re lucky enough to have a £50 note in your wallet or purse, you can have a look at how it turned out for yourself!
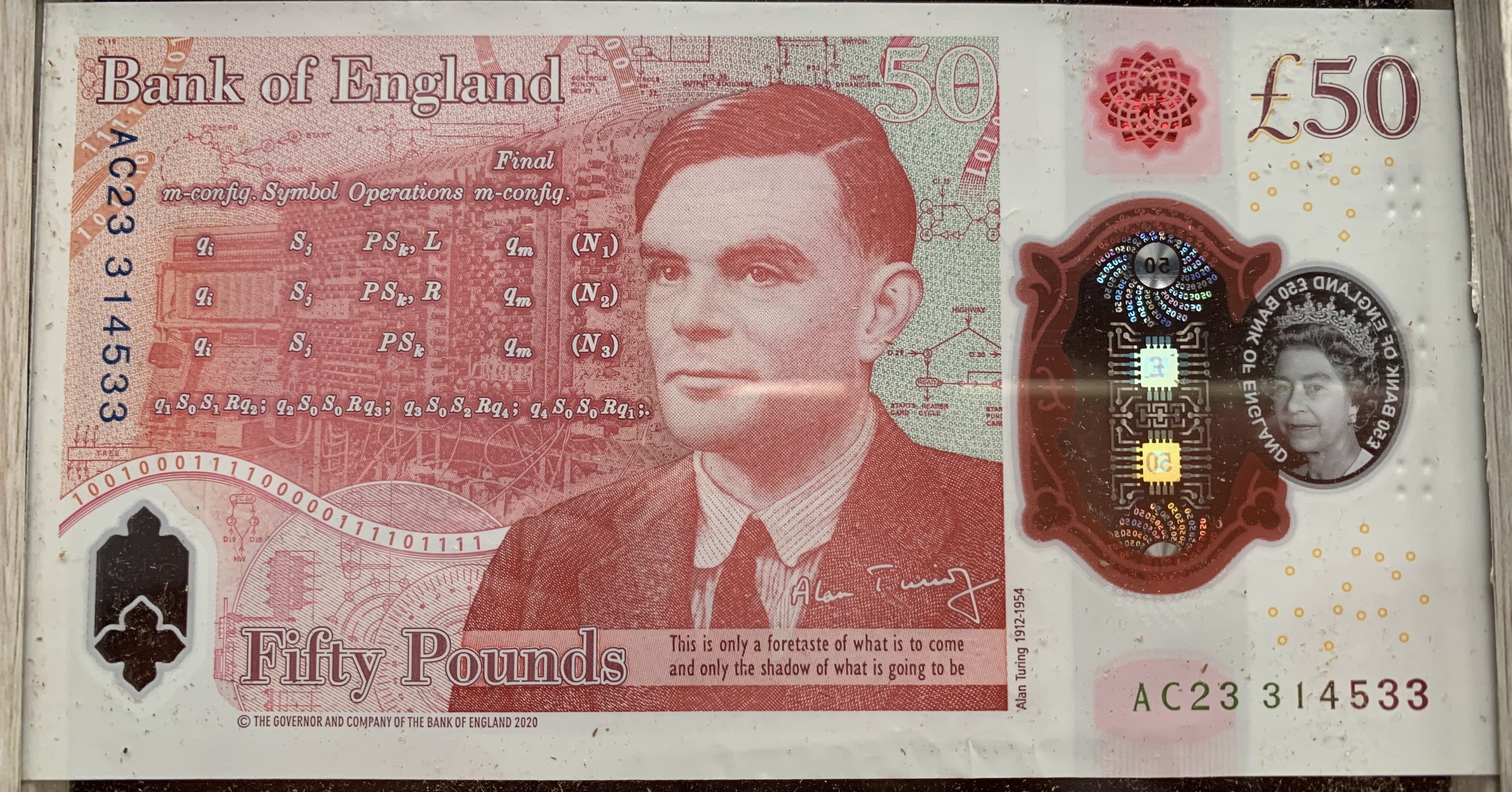
This website represents Arthur’s personal views.